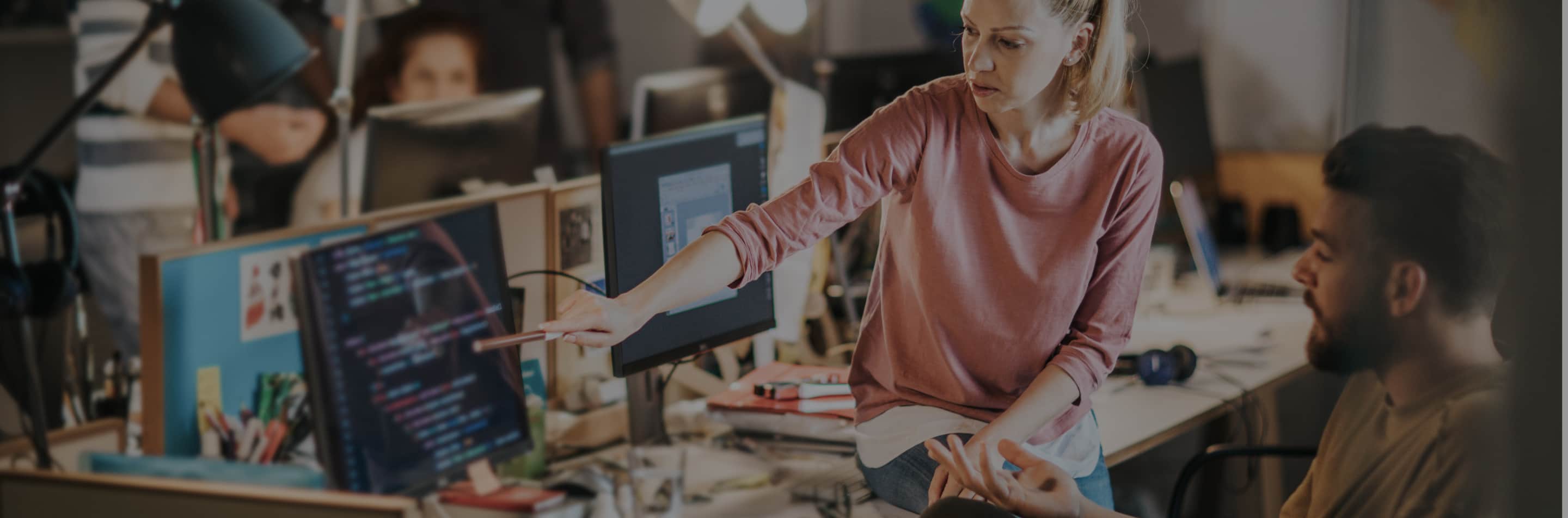
Case Studies
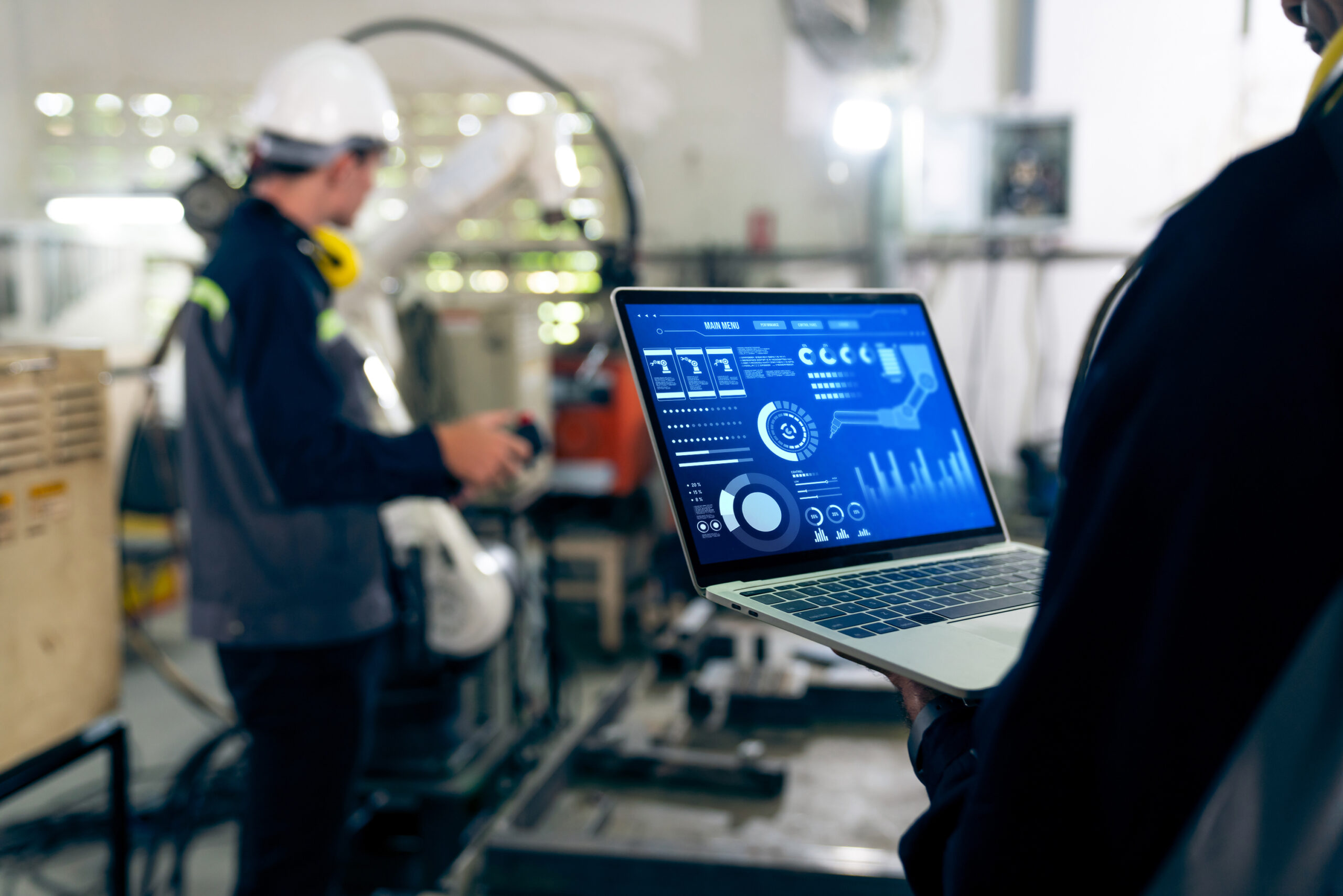
Implementing Predictive Maintenance in Industrial Automation
Context
Our client, operating in the dynamic sector of manufacturing, was contending with a critical industry-wide challenge: the unpredictability and frequency of machinery failures. These failures were not just routine technical hitches but complex breakdowns stemming from a myriad of factors such as wear and tear, operational stress, and undetected faults in system components. This unpredictability led to substantial unplanned downtime, severely disrupting production schedules.
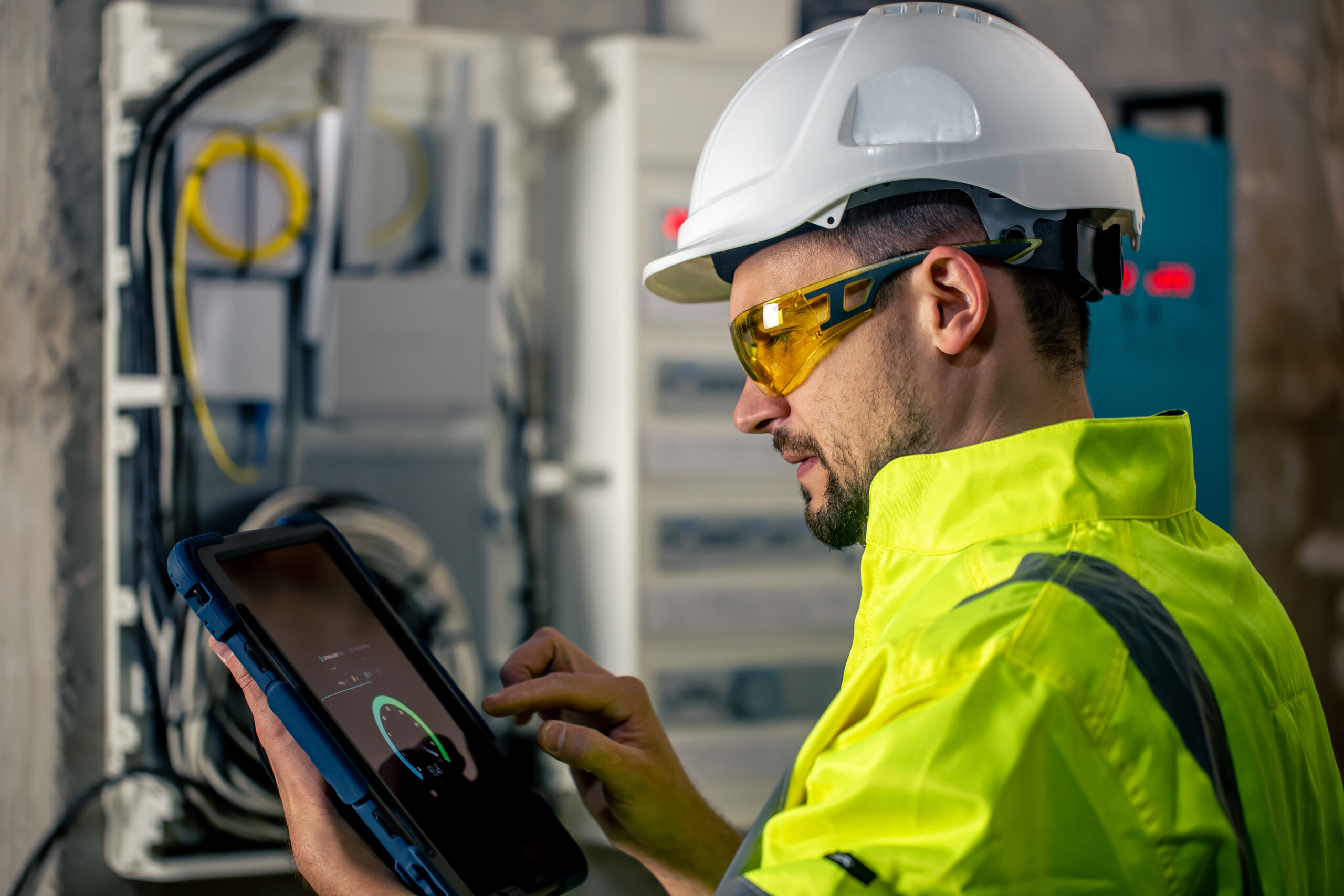
Reframing the Problem
In addressing the challenge of industrial machinery maintenance, we identified a need to shift our approach from a reactive stance to a more proactive, predictive model. The core of this redefined problem involves developing an embedded system capable of not only monitoring but also analyzing the performance of industrial machinery in real-time. This reframed perspective helped in creating a solution to preemptively identify potential issues, thereby optimizing machinery function and mitigating downtime.
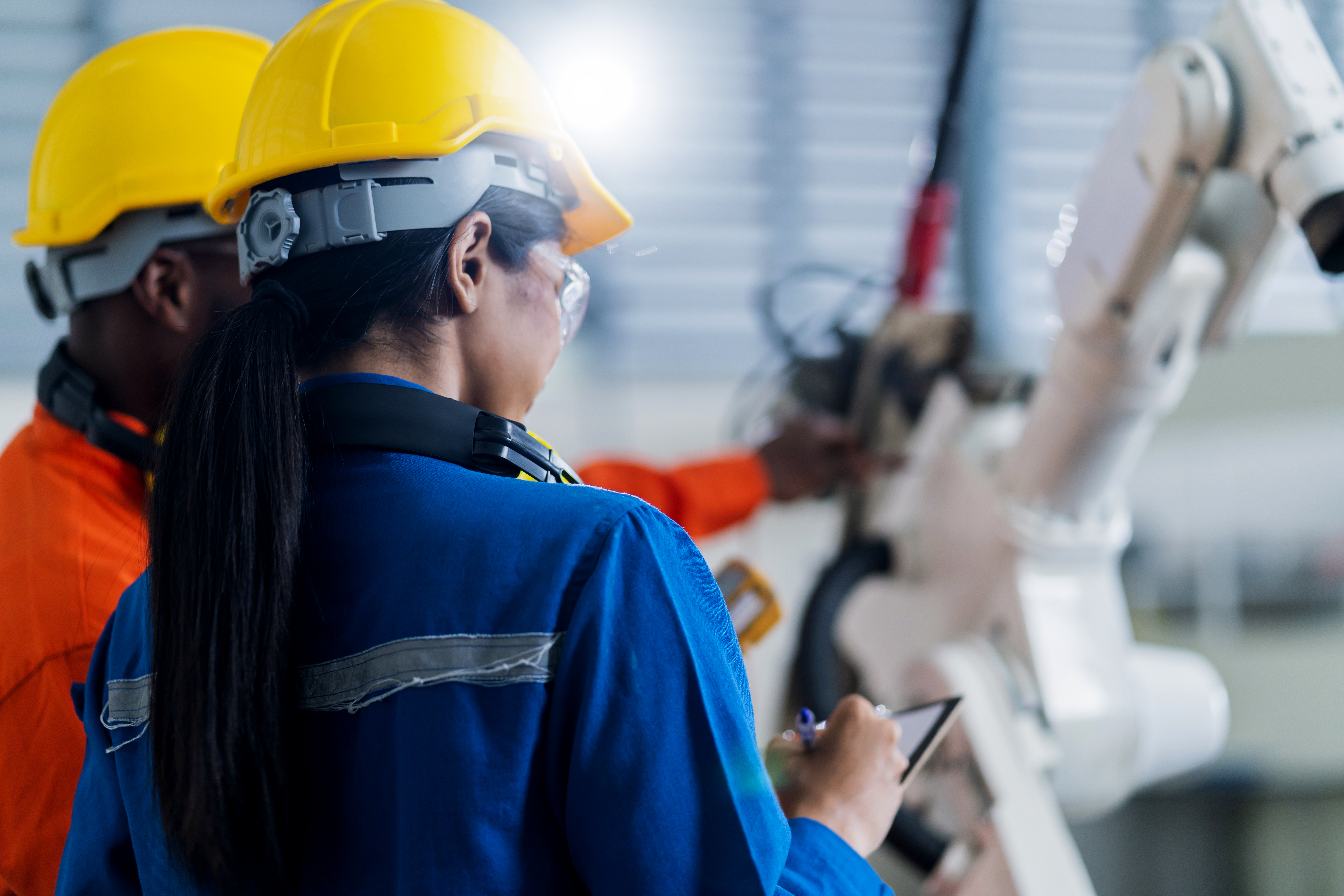
Our approach
1. Integration of Diverse Sensors: Alongside the ESP32 controllers, we deployed a variety of sensors tailored to the specific requirements of industrial machinery.
- Temperature Sensors like thermocouples for their wide range and durability and RTDs for high accuracy and stability were used.
- Vibration Sensors like Accelerometers and piezoelectric sensors were used for their sensitivity to vibration and pressure changes.
- Current Sensors like INA219 shunt resistor-based sensors were chosen for their high accuracy in current measurement, crucial for identifying electrical anomalies in machinery. The INA219 sensor’s high-precision measurements, coupled with its digital output and programmable calibration, played a critical role in the accurate monitoring of machinery performance by comparing the reading with OEM data.
2. Data Analytics and Predictive Algorithms: We developed proprietary algorithms to analyze the data from these sensors. By comparing real-time readings against established OEM performance curves, our system could predict potential machinery faults before they lead to breakdowns.
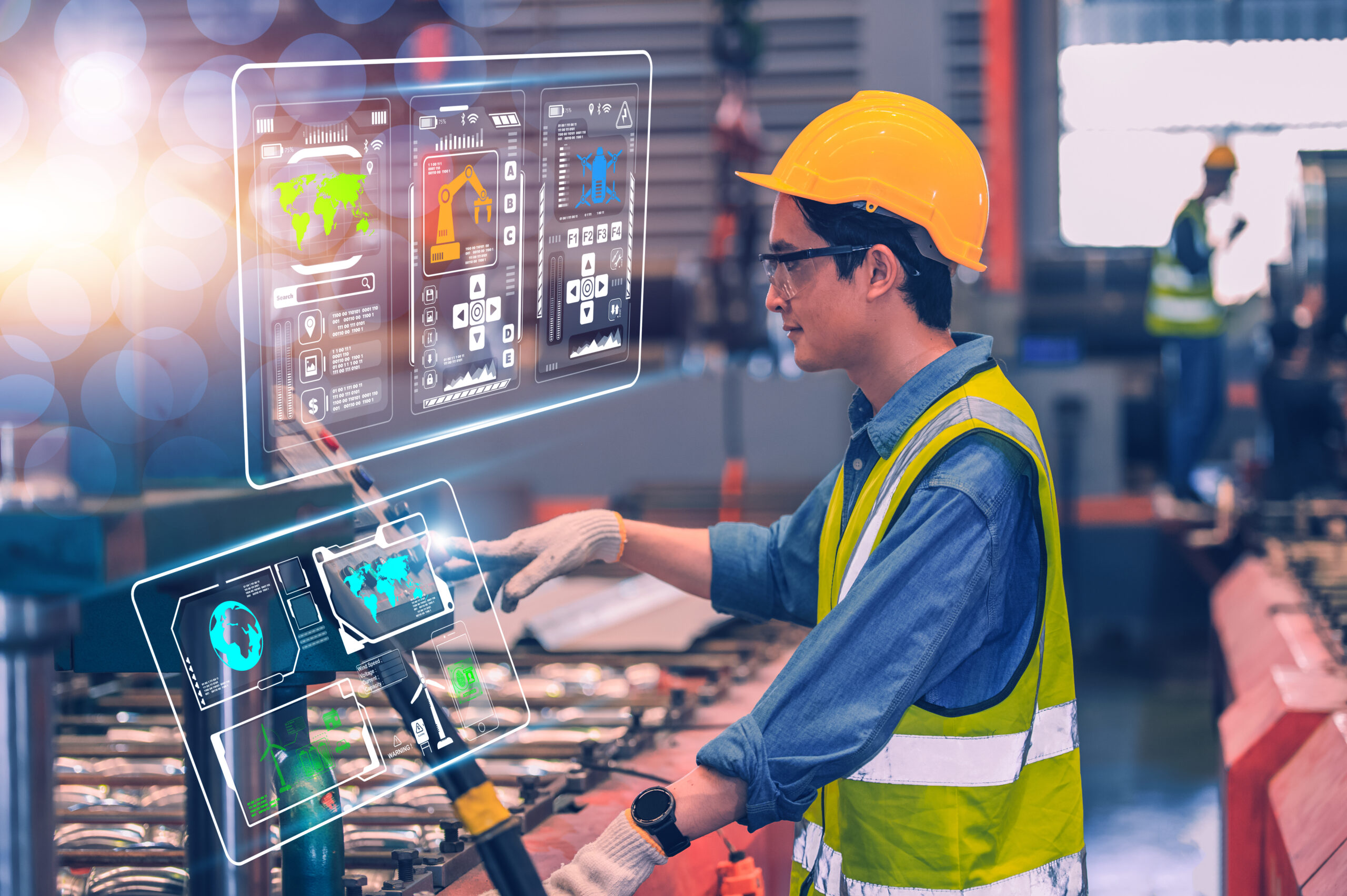
The Impact
1. Reduction in Unplanned Downtime: The adoption of the predictive maintenance system led to a significant decrease in unplanned machinery failures. This downtime reduction improved immediate productivity and created a more stable and predictable production schedule.
2. Optimization of Periodic Maintenance Schedules: One of the most notable impacts was on the annual and other periodic maintenance schedules. With the predictive insights provided by our system, the company could strategically plan maintenance activities during less disruptive periods, thereby minimizing the impact on production cycles.
3. Cost-effective maintenance and Increased Equipment Longevity: The precise monitoring and early fault detection allowed for targeted maintenance interventions. This approach prevented minor issues from escalating into major repairs, extending the lifespan of machinery and leading to substantial cost savings over time.
4. Overall Operational Efficiency: The integration of advanced sensor technology and predictive analytics not only enhanced the machinery’s performance but also played a crucial role in optimizing resource allocation and workforce for maintenance activities. This holistic improvement in maintenance operations directly bolstered the firm’s operational efficiency and bottom line.